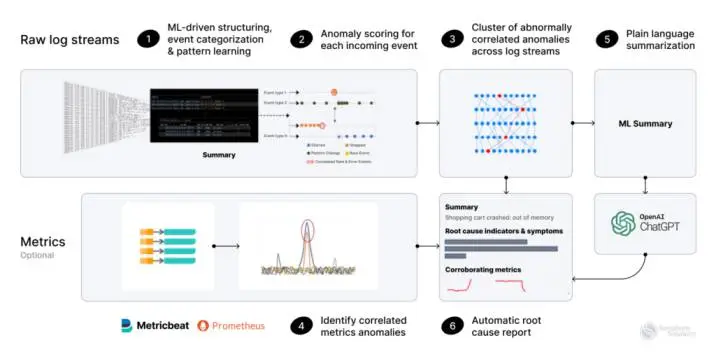
Key Benefits of Using Google Cloud for Machine Learning
Cloud ML Motor is an incredible asset given by Google Cloud Stage (GCP) that offers a scope of advantages for preparing and serving AI (ML) models.
By utilizing the capacities of Cloud ML Motor, clients can exploit a versatile and oversaw climate that improves on the method involved with building, preparing, and conveying ML models. In this response, we will investigate the different advantages of utilizing Google Cloud for Machine Learning and how it improves the ML work process.
One of the vital benefits of Google Cloud for Machine Learning is its capacity to deal with enormous scope ML jobs proficiently. With Cloud ML Motor, clients can prepare models on appropriated framework, which speeds up the preparation interaction by parallelizing calculations across different machines.
This circulated preparing ability takes into account quicker model emphasis and diminished opportunity to-arrangement. By exploiting the adaptability presented by Cloud ML Motor, clients can prepare models on enormous datasets without agonizing over asset impediments.
One more advantage of utilizing Google Cloud for Machine Learning is its reconciliation with other GCP administrations. Cloud ML Motor flawlessly coordinates with other GCP instruments, for example, Google Distributed storage, BigQuery, and Dataflow, permitting clients to access and handle information from different sources without any problem.
For instance, clients can store their preparation information in Distributed storage and straightforwardly train models involving that information in Cloud ML Motor. This reconciliation improves on the ML work process and takes out the requirement for manual information move or preprocessing.
Cloud ML Motor likewise gives a scope of pre-constructed ML calculations and structures, for example, TensorFlow, scikit-learn, and XGBoost. These pre-fabricated calculations offer an extensive variety of usefulness, permitting clients to rapidly construct and train models without carrying out complex calculations without any preparation.
Moreover, Cloud ML Motor backings custom holders, empowering clients to bring their own ML structures and libraries for preparing and serving models. This adaptability permits clients to work with their favored ML instruments while as yet profiting from the oversaw foundation given by Cloud ML Motor.
The arrangement of ML models is made simple with Cloud ML Motor's serving usefulness. When a model is prepared, it tends to be sent as a web administration, making it open for expectations from anyplace.
Cloud ML Motor consequently handles the scaling and burden adjusting of the conveyed models, guaranteeing high accessibility and low idleness for serving expectations. This capacity is especially helpful for applications that call for continuous expectations, like proposal frameworks or misrepresentation recognition.
Notwithstanding the specialized advantages, Cloud ML Motor additionally offers cost advancement highlights. With Cloud ML Motor, clients just compensation for the assets they consume during preparing and serving.
The programmed scaling and asset assignment capacities of Cloud ML Motor assist with advancing expenses by powerfully changing the assets in view of the responsibility. This guarantees that clients are not overprovisioning assets and just compensation for what they really use.
To sum up, involving Cloud ML Motor for preparing and serving ML models carries a few advantages to clients. These incorporate effective treatment of enormous scope ML jobs, consistent coordination with other GCP administrations, support for pre-fabricated ML calculations and custom compartments.
Simple arrangement of models as web administrations, and cost enhancement highlights. By utilizing these advantages, clients can speed up their ML work processes, decrease functional intricacy, and accomplish better versatility and cost proficiency.
Google Cloud & Machine Learning: An Overview
With the sheer volume of information that most present day organizations are burdened with, it very well may be hard to play out a legitimate investigation utilizing customary business insight instruments. Thus, many are going to AI to push their business forward.
AI models need far reaching registering and stockpiling capacities to perform, and business associations need an available, savvy answer for fabricate their AI programs.
Google Bard: The Next Step in AI
Notwithstanding the numerous simulated intelligence and ML cloud administrations given by Google Cloud Control center, Google is likewise blasting another way ahead for computerized reasoning with its progressive conversational artificial intelligence innovation, Troubadour. Google Poet is a man-made intelligence controlled chatbot created by Google that responds to your inquiries with strikingly human reactions.
Poet easily incorporates data from the web to give the freshest, best outcomes. The chatbot can perform text-based assignments like making different types of composed content, summing up text, and interpreting between dialects, among different abilities.
Clients just sort their brief into the text box and press enter to start a discussion with Versifier. The chatbot's reactions are separated into absorbable organizations, making it simple to check and fathom.
With its regular language handling capacities, Troubadour can comprehend and answer prompts in a conversational way, making it a brilliant examination device and imaginative teammate.
What is Bard Based On?
Right away, Versifier could appear to be basically the same as ChatGPT. All things considered, they're both computer based intelligence chatbot administrations intended to answer a wide range of inquiries. In any case, it's vital to take note of that there are contrasts between these two advances.
ChatGPT and Minstrel are both Enormous Language Model (LLM) based chatbots that utilization AI and Regular Language Handling (NLP) innovation to produce reactions in light of the prompts you give them.
The fundamental contrast between the two is their design. ChatGPT depends on OpenAI's GPT-3, while Poet utilizes Google's LaMDA innovation. LaMDA was prepared on conversational exchange to figure out the particular importance and goal behind prompts, while ChatGPT was prepared on textdatabases from the web, including books, webtexts, wikis, and articles.
Getting Started with Google Cloud for Machine Learning
In the event that your group is thinking about utilizing Google Cloud for AI, reach out to the Google specialists at Primero. We'll work with you to comprehend your particular AI needs and foster a custom answer for mitigate your most squeezing trouble spots.